The Role of Generative AI in Testing of Digital Health Products
|
Introduction
Amidst the swiftly changing and highly competitive realm of digital health and health tech, it is crucial to prioritize the quality and dependability of products. Within this domain, QA and testing professionals encounter many challenges, ranging from data management and adhering to HIPAA regulations to dealing with interoperability issues and complex integrations. Nonetheless, the advent of generative artificial intelligence, commonly known as Gen AI, presents an opportunity for teams to carry out testing and QA procedures with enhanced effectiveness and efficiency.
Ensuring quality and reliability is paramount in the fast-paced world of digital health products. Quality assurance and testing are crucial in identifying and rectifying defects, optimizing performance, and enhancing user experience. The quality assurance and testing landscape has transformed with the emergence of generative artificial intelligence (AI) technologies. This article explores the role of generative AI in the quality assurance and testing of digital health products, delves into the challenges associated with its implementation, and provides strategies to overcome them.
Generative AI in Quality Assurance and Testing
Overview of Generative AI
Generative AI is a subset of artificial intelligence that focuses on creating models capable of generating new data or content. It encompasses various techniques such as generative adversarial networks (GANs), deep reinforcement learning, and variational autoencoders. These technologies enable machines to learn patterns from existing data and generate new, realistic data based on those patterns.
Applications of Generative AI in Quality Assurance and Testing
- Test Data Generation: Generative AI can generate diverse and representative test datasets, encompassing various scenarios, edge cases, and real-world user data. This ensures comprehensive test coverage and reduces the risk of overlooking critical scenarios.
- Test Case Generation: Generative AI algorithms can automatically generate test cases by analyzing software requirements, code, and existing test suites. This streamlines the test case creation process and improves efficiency.
- Bug Detection and Prediction: Generative AI algorithms can detect and predict potential bugs or defects by analyzing patterns in software code and testing data. This enables early bug identification and faster debugging, reducing the likelihood of releasing flawed products.
- Performance Testing: Generative AI techniques can simulate realistic user behavior and workload patterns to conduct performance testing. This helps assess system scalability, response times, and resource utilization under various conditions.
Benefits of Using Generative AI in Quality Assurance and Testing
- Increased Efficiency and Scalability: Generative AI automates various testing processes, significantly reducing manual effort and time. It enables testing teams to handle complex scenarios and large datasets, enhancing scalability efficiently.
- Improved Test Coverage: Generative AI generates diverse test data and cases, enabling comprehensive coverage of scenarios, inputs, and edge cases. This minimizes the risk of missing critical issues during testing.
- Faster Bug Detection and Resolution: Generative AI algorithms can identify potential bugs and defects early in the development cycle, facilitating quicker debugging and resolution. This accelerates the overall development process.
- Enhanced Performance Testing: With generative AI, performance testing becomes more accurate and realistic, simulating real-world usage patterns. This ensures that digital health products can handle expected user loads without degradation.
Challenges in Implementing Generative AI in Quality Assurance and Testing
Data Availability and Privacy Concerns
Implementing generative AI techniques in quality assurance and testing requires access to relevant and representative data. However, acquiring such data can be challenging due to privacy concerns, legal restrictions, and limited availability. Ensuring compliance with data protection regulations and establishing robust data management processes is crucial.
Training and Fine-Tuning of AI Models
Generative AI models must be trained on large datasets to learn patterns and generate accurate outputs. However, acquiring labeled data for training can be time-consuming and resource-intensive. Additionally, fine-tuning the models to suit specific testing requirements and domains may require specialized expertise.
Interpretability and Explainability of AI Results
Generative AI algorithms often produce outputs that may be difficult to interpret or explain, making it challenging for testers and developers to understand the underlying reasoning. This lack of interpretability raises concerns about the reliability and trustworthiness of AI-generated results.
Ethical Considerations in AI Testing
Using generative AI in testing raises ethical concerns, such as bias in data, discriminatory outputs, or unintended consequences. Fairness, transparency, and ethical guidelines become essential to prevent any adverse impact on the end-users or vulnerable populations.
Adoption and Integration Challenges
Integrating generative AI techniques into existing quality assurance and testing processes may require significant infrastructure, tools, and skill sets changes. The need for more awareness and understanding of generative AI among testing teams can hinder its adoption and effective utilization.
Strategies to Overcome Challenges
Data Management and Privacy Measures
Organizations should establish data governance frameworks to address data availability and privacy concerns, ensure compliance with relevant regulations, and explore alternative approaches such as synthetic data generation. Anonymization and encryption techniques can also be employed to protect sensitive data.
Robust Training and Fine-Tuning Processes
Organizations can collaborate with data scientists, leverage pre-trained models, and employ transfer learning techniques to mitigate the challenges of training and fine-tuning AI models. Data augmentation and active learning approaches can help generate labeled data more efficiently.
Ensuring Interpretability and Explainability
Organizations should focus on developing AI models and algorithms that provide interpretable and explainable outputs. Techniques such as rule-based post-processing, visualization, and model-agnostic explanations can enhance transparency and enable a better understanding of AI-generated results.
Ethical Guidelines for AI Testing
Implementing ethical guidelines for AI testing helps mitigate potential biases, ensures fairness, and minimizes user harm. Organizations should establish clear protocols for data collection, bias detection and mitigation, and regular auditing of AI models to ensure ethical standards are met.
Smooth Adoption and Integration
To facilitate the adoption of generative AI techniques, organizations should provide training and education to testing teams, foster collaboration between testers and data scientists, and gradually integrate generative AI into existing testing workflows. Pilot projects and proofs-of-concept can help demonstrate the value and benefits of generative AI.
Case Studies of Generative AI in Quality Assurance and Testing
Real-world Examples of Generative AI Implementation
Illustrate case studies where generative AI has been successfully applied in digital health product quality assurance and testing. Highlight the challenges addressed, the outcomes achieved, and the impact on product quality and reliability.
Benefits and Outcomes of Generative AI in Quality Assurance and Testing
Discuss the tangible benefits experienced by organizations that have implemented generative AI in their quality assurance and testing processes. These may include improved defect detection rates, accelerated testing cycles, enhanced user experience, and increased customer satisfaction.
Future Directions and Opportunities
Advances in Generative AI and its Impact on Testing
Explore the potential advancements in generative AI techniques, such as self-supervised learning, active generative models, and unsupervised anomaly detection, and discuss their potential impact on quality assurance and testing in the digital health industry.
Integration of Generative AI with Other Testing Techniques
Highlight the synergies between generative AI and other testing techniques such as automation, exploratory, and security testing. Discuss how combining these approaches can lead to more comprehensive and effective quality assurance practices.
Importance of Continuous Learning and Adaptation
Emphasize the need for organizations to stay updated with the latest developments in generative AI and continuously adapt their testing strategies. Encourage a culture of continuous learning, experimentation, and knowledge sharing among testing teams.
Conclusion
In conclusion, generative AI has emerged as a powerful tool in the quality assurance and testing of digital health products. Its applications, such as test data generation, test case creation, bug detection, and performance testing, have demonstrated significant benefits, including increased efficiency, improved test coverage, faster bug detection, and enhanced performance testing. However, implementing generative AI in quality assurance and testing has its challenges.
The challenges include data availability and privacy concerns, training and fine-tuning of AI models, interpretability and explainability of AI results, ethical considerations, and adoption and integration challenges. To overcome these challenges, organizations can employ various strategies.
Effective data management and privacy measures, such as data governance frameworks and anonymization techniques, can address data availability and privacy concerns. Collaboration with data scientists, leveraging pre-trained models, and employing transfer learning techniques can help mitigate the challenges of training and fine-tuning AI models.
To ensure interpretability and explainability, organizations should focus on developing AI models and algorithms that provide transparent outputs. Techniques like rule-based post-processing, visualization, and model-agnostic explanations can enhance understanding of AI-generated results.
Implementing ethical guidelines for AI testing is crucial to prevent biases, ensure fairness, and minimize harm to users. Clear protocols for data collection, bias detection, mitigation, and regular auditing of AI models can help uphold ethical standards.
Smooth adoption and integration of generative AI techniques require training and education for testing teams, collaboration between testers and data scientists, and gradual integration into existing workflows. Pilot projects and proofs-of-concept can demonstrate the value and benefits of generative AI in quality assurance and testing.
Real-world case studies of generative AI implementation in quality assurance and testing can provide tangible examples of its benefits. These case studies can showcase improved defect detection rates, accelerated testing cycles, enhanced user experience, and increased customer satisfaction.
Looking to the future, advances in generative AI techniques, such as self-supervised learning and unsupervised anomaly detection, hold promise for further improving quality assurance and testing in the digital health industry. Integrating generative AI with other testing techniques, such as automation and security testing, can lead to more comprehensive and effective quality assurance practices.
In conclusion, generative AI has revolutionized digital health product quality assurance and testing. While there are challenges in implementing this technology, organizations can overcome them through effective strategies. By harnessing the power of generative AI, digital health companies can enhance their products’ quality, reliability, and performance, ultimately benefiting both patients and healthcare providers.
Achieve More Than 90% Test Automation | |
Step by Step Walkthroughs and Help | |
14 Day Free Trial, Cancel Anytime |
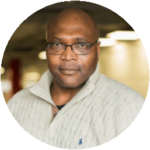